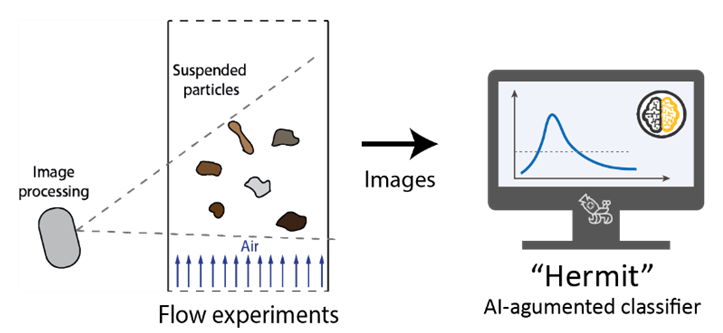
AI-supported investigation of the turbulence-granulate interaction
- Contact:
- Project Group:
Machine intelligence in energy systems
Overview
Due to the growing population and an expanding global economy, the amount of waste produced worldwide is constantly increasing. Much of this waste is still disposed of in landfills and incinerated instead of being processed and reused. In order to separate the individual materials efficiently (e.g. in a fluidized bed separator), detailed knowledge of the gas-solid interactions of these complex granular mixtures is essential. Therefore, there is a need for an "expert system" that can classify particles and simultaneously extract the orientation information for a polydisperse, multiform particle flow with "minimal monitoring".
The Institute of Thermal Fluid Machinery is therefore investigating the extent to which a deep learning model trained using synthetic data and specialized in object recognition is able to extract the shape and orientation tendencies of arbitrarily shaped particles. The decisive factor here is to generate a training dataset with images of different particles that are as real as possible and for which the shape and orientation are known. On the one hand, this can be achieved by mapping real particles as accurately as possible in a virtual world, which can then be used to generate any synthetic training data.On the other hand, this can be achieved by using real images of particles that are automatically labeled with the aid of synthetic data and can then be used as training data.
The aim of the project is therefore to develop an AI-based platform to solve this image classification and orientation trend problem for the waste separation process in the context of the zero waste cycle. This interface has the potential to help solve the problem of turbulence-granule interactions in a proactive way by exploring the underlying dynamics and extracting explicit correlations - an important strategic step towards achieving the goal of zero waste cities.
Challenges
- Generating a sufficiently large training dataset using synthetic data
- Identifying individual particles in the image using an object recognition model or other particle tracking methods
- Extraction of particle shape and orientation trends from a time series of images taken from different positions
- Validation of the platform using real particle flows in the experiment