AI-Augmented Discovery of Turbulence-Granular Material Interactions for Sustainable Waste Management
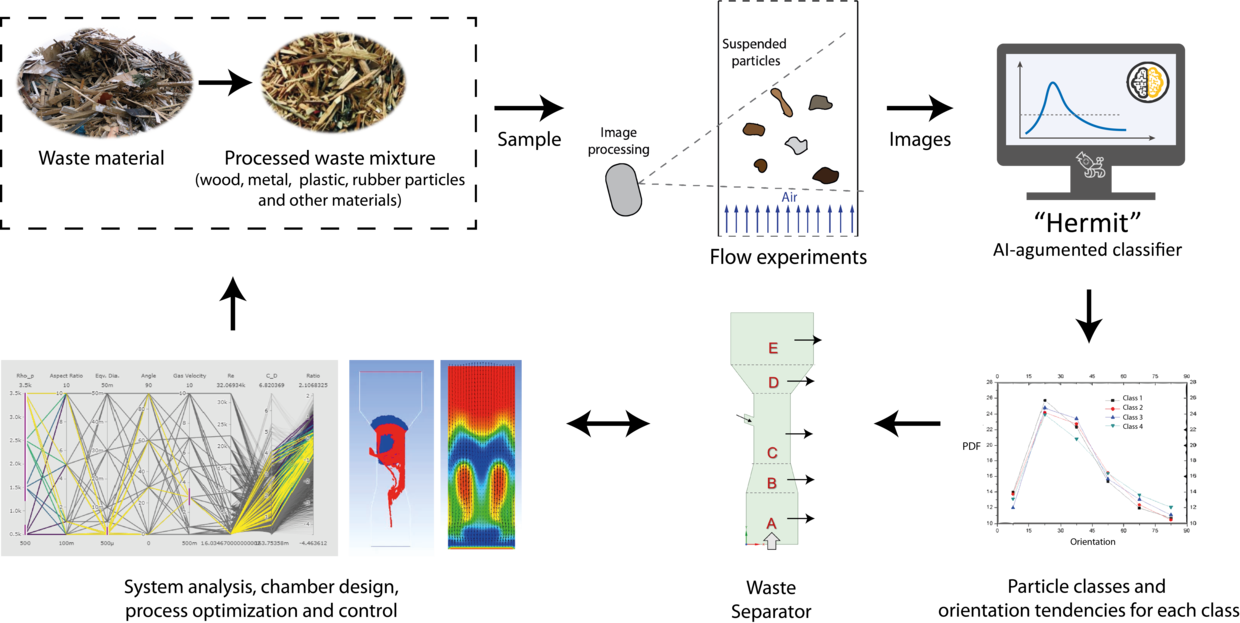
Growing population, expanding global economy, urbanization with rising standard of living have dangerously accelerated waste generation in the world. It is expected to reach over 3.4 billion tons of waste per year by 2050, roughly a 70% increase. One such goal involves separating the waste mix into different fractions, such as recyclable wood, metal, glass, and plastic, which in turn provides tremendous environmental benefits in terms of virgin material substitution, avoided energy demand, greenhouse gas emissions reduction, and water footprint. An unmet requirement for this technology is a thorough understanding of the principles that govern gas-solid interactions for these very complex granular mixtures. Thus, there is a need for an "expert system" that can perform particle classification while extracting orientation information for a polydisperse, multiform particle cloud with "minimal monitoring."
In this project, the primary goal is to develop an AI-based platform to solve this image classification and orientation tendency problem for the waste separation process in the "zero waste" cycle. The platform will leverage two of the most triumphant implementations of narrow AI, image processing and generative learning, to accomplish both tasks. Following the successful demonstration of the developed particle field information extraction platform, the next step is to synchronize the model with a symbolic model and upgrade the platform to a "Neuro-Symbolic Particle Analyser" for the discovery of turbulence-granule interactions. This interface has the potential to help solve the problem of turbulence-granule interactions in a proactive manner by allowing us to explore the underlying dynamics and extract explicit correlations - an important strategic step towards achieving the goal of "zero waste cities".